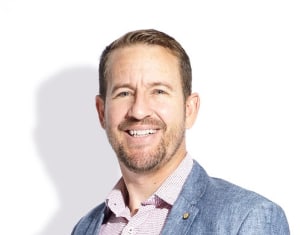
AI is on the tip of everyone’s tongue but is it at their fingertips too, asks Mindshare’s MD of Digital Solutions Daniel Benton, offering ways to ensure everyone is getting started and fuelling progress.
What starts in science fiction is increasingly becoming the reality we all live in. AI is no different. Acclaimed sci-fi author William Gibson was at the forefront. Arguably his most famous line “the future is here but it’s not evenly distributed” is highly applicable for the current state of artificial intelligence in the media and marketing industry.
While AI is likely the most hyped class of technology today, I also agree with Mustafa Suleyman (formerly of DeepMind, now star Microsoft hire and author of the brilliant “The Coming Wave”) that AI is “general purpose” in nature and will be transformative across broad swathes of society. Including the media and marketing industry.
So where are we today?
While the launch of Chat GPT in late 2022 was seismic, AI (particularly Artificial Narrow Intelligence) products have been in general availability for years prior, both in our daily lives and in specific marketing and media use case, think;
- Google maps mapping models
- Content recommendation algorithms from Spotify, Netflix, YouTube, TikTok etc
- Dynamic ad inventory (Google Ads)
- Objective and model based bidding (Google Ads, Meta etc)
- Narrative sciences automated data narratives
With AI computing power growing at 1000% annually (according to OpenAI) these products will only continue to rapidly evolve in sophistication and be joined by newer solutions e.g.:
- Adobes injection of AI features into its creative cloud.
- Googles push to migrate advertisers into heavily automated ad solutions like demand gen and p-max (more on this later).
- Open AI’S Sora text to video generation model
So with this rapid growth in sophistication Is the answer always AI?
Short answer no. In my experience AI solutions aren’t always the answer (despite the hype) and need to be evaluated critically before testing them – particularly if the test is with someone else’s budget. The questions that need to be answered are;
- Is it really AI? There are a lot of products using boolean “if this, then that” logic models that have been badged as AI to up the sizzle factor.
- Is AI really needed for this task / use case? Is there a simpler lower tech / lower cost solution?
- Is there inherent survivor bias in the case studies selling the AI solution? When didn’t the solution work and why?
- Is the underlying model clearly aligned with what you’re trying to achieve? An example of this is Google’s p-max, which while I’m sure delivers excellent results for some advertisers can have some unintended consequences.
Beyond these questions, I think it’s incumbent on all of us to try and improve our individual and teams’ critical thinking and logic skills to ensure we’re making the best possible decisions in environments of increasing complexity.
What are the actual practical use-cases?
And when AI is the answer, the main short term wins that AI can unlock for all of us today are in the “1 percenter” tasks that will enable you or your team to run faster or get to things that previously weren’t feasible e.g.:
- Targeted document summary
- Transcription and meeting summaries
- Sub-editing, rewriting and structuring content
- Visual concept ideation
- Custom Image generation
- Data analysis, classification and visualisation
- Asset classification and tagging
The way I think about using AI with these tasks is it’s not about replacing a human doing the work, it’s more about either compressing time or extending capability. For example a human could classify thousands of creative assets with descriptive metadata following a clear naming convention. But would they want to do it? And if they did what would the error rate be? And how long would it take?
There is however one wrinkle in using AI for these and other tasks. The user needs to have the pre-existing foundational skills and experience to know what “good looks like”. Without these skills and an overreliance on an AI model it’s possible to end up with outputs that appear plausible but are wholly incorrect. This means as an industry we will need to balance creating environments where junior talent can build foundational skills and experience while also increasingly using AI to create efficiencies. The ideal scenario is where AI outputs are critiqued, validated and calibrated via sample testing from end users – which will take some thoughtful consideration to engineer.
We’ve found some innovative ways ahead by empowering our teams with WPP Open, our AI-powered intelligent marketing operating system. Built on data sources and LLMs it enables us optimise the entire marketing process, it helps us optimise for effectiveness, push and test ideas as well as increase productivity through reducing manual tasks.
There are some other key considerations before jumping into testing AI for these and other tasks;
- For any sensitive tasks AI / ML models need to be hosted in secure partitioned environments, so any data ingested isn’t exposed externally to train larger models.
- Unforeseen future legal risks should be considered, particularly around the use of AI models that have been trained on unlicensed copyrighted material. Which is why I think brands will ultimately end up training bespoke models with their own ip protected content.
- Model overconfidence; understanding the limitation / blind spots of models prior to exposing tools to the wider organisation is critical.
Where to from here?
It’s safe to assume the pace of technical evolution is unlikely to slow down with AI being embedded in more of the processes and tools we use day to day. But it's not a one-size-fits-all approach. Our industry is grappling with the rapid advancements in AI, from synthetic research, content recommendation algorithms to automated ad solutions. While the potential benefits are myriad, a critical and discerning approach is essential. AI solutions need to be evaluated based on their underlying models, ideal use cases, and potential biases.
There are some basics that individuals and organisations can tackle to get started.
- Push to get access to the tools. The free version of chat GPT is a good starting point for cheap R&D – but only with non-sensitive data.
- Have a framework to identify and prioritise use cases to pilot. Ideally all use cases have baseline metrics to allow the quantification of improvements from an AI model.
- Run the pilot and document the learnings (model used, prompts used, workflows, pitfalls etc)
- Assess the pilot performance against the initial metrics. Operationalise the winners and share the learnings of the failures across the organisation.
This should mean we’re better placed to both unlock the potential of the technology and identify for blind spots. However, the uneven distribution of AI's impact means that some organizations and teams will be better positioned to benefit from its capabilities than others. The key is to strike a balance between creating an environment where all team members are encouraged to safely experiment and build foundational skills while also ensuring there are guard rails and leadership to drive the strategy and mitigate for ethical and legal downsides.
Getting this right will mean that organisations can ensure the AI future is not just here, but evenly distributed, empowering media and marketing professionals to drive innovation and growth in a responsible and sustainable manner.
Daniel Benton is Mindshare Managing Director of Digital Solutions