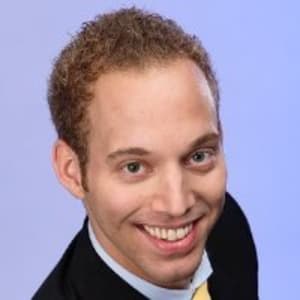
Attribution modelling has garnered much attention among marketers. And there are good reasons for this buzz. The goal of attribution models is to understand how media drive sales or other important performance measures.
This is a problem that has kept CMOs and media planners busy for a long time. However, attribution modelling is more than 'just another way' to measure advertising effectiveness. Traditionally, questions revolving around the impact of marketing channels (TV, radio, digital etc.) have been answered using aggregate data, such as weekly marketing spend per channel, and complicated statistical models for time series. This approach is often referred to as market response modelling or marketing mix modelling.
In contrast to marketing mix models, attribution models are based on individual consumers' advertising exposures. Until recently such individual-level data were available only through relatively expensive single-source panels. But nowadays we can access information on all consumer touchpoints with a brand or product through site analytics and ad-server data – the backbone of attribution modelling. All that is needed are tags – little pieces of computer code on websites – for each activity and event one wishes to track and include in the model.
Possessing data on each individual customer and their journey has tremendous advantages. Such analyses tend to be more accurate and robust than those using aggregate data, where we need to assume that all consumers behave in the same way.
Furthermore, attribution models based on algorithmic methods reduce classical perils in statistical analyses: the risks to confound variables or the cause and effect are much smaller. There are also huge differences in the time period required to carry out any analysis at all. For the traditional analyses (using aggregate spend per week), one typically needs about one to two years of data to obtain reliable insights.
However, two weeks of data on consumer touchpoints can be sufficient to get first results with attribution modelling. And attribution models can be updated daily – these timing differences can create a big strategic advantage for any media planner who needs to react quickly to the changes in the fast-paced world of digital and automated marketing.
Apart from benefits due to higher accuracy and better timing, the granularity and richness of consumer-advertising data allows light to be shed on questions that were difficult to tackle before.
With attribution models, one can examine how much single placements, tactics, or even creatives, influence consumers. Because we can see all consumer touchpoints in their journey to the purchase or conversion, we can now look at the relevance of sequence of events.
For example, what does the typical path to conversion look like? Which ads drive consumers in the lower purchase funnel? Which media combinations help move consumers beyond brand awareness towards engaging in a brand?
In addition, attribution models can provide new insights into customer loyalty and the cross-selling impact of media.
We can see which customers repeatedly convert and in which order they do so. Do they first look for a retail location and then download brochures, or vice versa?
We can also investigate which advertising is most efficient for different segments. For example, which media and tactics (keyword or audience retargeting) work best for repeat and first-time converters. Similarly, some companies may have related brands or campaigns.
Attribution models can easily be extended to analyse how consumers are affected by advertising of different product lines from the same brand.
Testing so many factors and their interplay in one model would be nearly impossible with traditional statistical models based on aggregate data or with other methods (e.g., field experiments or A/B testing). Of course, there will always be some important consumer decision drivers that are easier to investigate using time-series of aggregate data, such as seasonal influences or the role of competitor activities. So marketing mix modelling will certainly not disappear from the analytics landscape.
However, with the increase in digital measurements and the rise of programmatic TV and radio, we are likely to see much more attribution modelling in the future.
By Nico Neumann
Lead statistician - product development at Anomaly Research and Analytics (part of IPG Mediabrands)