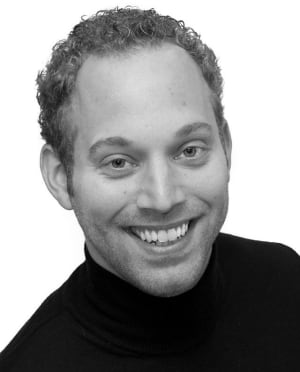
Digital advertising and ad tech companies are facing a lot of heat because of the ongoing discussions as to which ads are actually seen by consumers. Statistics vary, but it has been reported that around half of all ads could be fraudulent or not viewable. It is no surprise that many advertisers and brands appear upset. But do they really need to be so? What can clients do to ensure their money is not wasted?
Before condemning digital marketing, let’s be clear: there has been and always will be noise in data and no media metric is perfect. And this applies to offline media as well. For example, let’s recall how we tend to measure TV ratings.
One essential part of this process typically is the people meter, which captures how many household members are watching a particular TV program. It should be noted that panel households need to manually record the presence of each person using a remote control… constantly! So every time someone leaves or enters the room, even for some seconds, the panel member is supposed to push a button on the remote to track the number of people who are watching TV. This cumbersome procedure raises the question: how much trust can we have in panel members to do this every time? If one person from a two-person household is not in front of the TV while an ad is broadcasted, but forgets to adjust the people meter, we again have a 50% error rate for the tracked viewability of this ad. In addition, the panel attendance for all TV shows is statistically extrapolated to the population and any potential error may even be multiplied by thousands if not millions.
Unmistakably, we can discuss all possible forms of measurement standards, verifications and methodologies, but records of consumer ad exposures are likely to stay imperfect for all media types, no matter whether online or offline. Even if there was no measurement error in technical viewability, we do not know whether or not a person really paid attention to a particular ad on the TV, desktop or mobile screen. The bottom line is that we will have to live with measurement errors.
The good news is that the measurement issues related to advertising exposures do not need to be a major problem for clients – at least for those who make use of analytics. Why? Because your attribution or media mix analysis can take care of the problem (in terms of misspending). If a publisher or DSP sells a lot of unviewable ads (below the screen or served to bots), then their impact on your conversions must be low or zero because there cannot be a behavioural reaction from a consumer. Consequently, a well-working attribution model should suggest shifting money to providers who have better inventories or potentially to other marketing channels (e.g., SEM or TV). In other words, unviewable placements should be a primary concern for those who (re-)sell them and less for efficient media buyers.
Moreover, how much influence any media measurement error has on your budget allocations depends strongly on your attribution strategy. Firstly, it is generally more prudent to rely on end-of-the-funnel conversion metrics. In contrast, lower-funnel or engagement metrics, such as clicks, shares, downloads or likes, are problematic as they can be wrong (e.g., clicks occurring by chance) or manipulated with ease by a third party. Using lead generations may be a bit safer, but these metrics may still be prone to potential fraud (e.g., filling out forms with random names). Therefore, we all should aim to strictly model sales whenever possible, in particular, in times where we even have the technical abilities to link offline sales to online data.
Secondly, the way you calculate credit for conversions affects how much a measurement error based on false viewability can bias your strategic decisions. If you use data from converters only and simple heuristics, such as fixed-rule weighting of touchpoints or first-/ last-touch attribution, then your attribution results could be very wrong.
Imagine there is a cookie that happens to have an unviewable placement as the last event before a conversion. In case of last-touch attribution, which is still widely used, this ad would even get 100% of the credit even though it is impossible that this ad played any role in driving a conversion. Weighting rules may give such an ad less credit, but still way too much influence. The reason that all these simple rules fail here is because they neglect the additional long tail of cookies, devices or ad IDs that are likely to have unseen (and thus inefficient) ads in their event-data path. Yet, when using complete event data (on converters and non-converters) and sophisticated algorithmic models, then attribution analysis will reveal differences in media efficiencies, including wasted money due to ads that were not viewed by anyone.
Of course, being able to identify publishers or placements with low conversion rates does not eliminate the need to battle ad fraud and continuously improve our standards. Eventually, any advancement in measurements will also enhance the results of our media analyses.
However, given the persistence of some form of error for all advertising data, the most urgent task should be to find the medium or channel with the greatest ROI. Fortunately, every stakeholder (client, trading desk, DSP), who buys data from someone else in the ad tech supply chain, can leverage media attribution modelling to do so. The better their skills here, the more likely they will shine and outperform competition.
Want more from Nico? See below: